- Knowledge for Good at Meta is open-sourcing the information used to coach our AI-powered inhabitants maps.
- We’re hoping that researchers and different organizations world wide will be capable to leverage these instruments to help with a variety of initiatives together with these on local weather adaptation, public well being and catastrophe response.
- The dataset and code can be found now on GitHub.
To help the continuing work of researchers, governments, nonprofits, and humanitarians world wide, the Knowledge for Good at Meta program is open-sourcing the primary set of coaching information and pattern code used to assemble Meta’s AI-powered population maps.
Because the world appears in direction of the growing menace of local weather change, Meta’s AI-powered inhabitants maps, and the information behind them, supply vital alternatives to direct investments in catastrophe preparedness by improved estimation of global flood exposure and in climate adaptation planning.
By open sourcing these instruments, we hope that different researchers can generate new insights for rushing the supply of sustainable vitality and local weather resilient infrastructure world wide.
Why we’d like higher inhabitants maps
Correct estimates of inhabitants are taken without any consideration in lots of nations. Governments in superior economies can depend on quite a lot of sources together with tax information or census datasets to raised estimate their inhabitants and make knowledgeable selections on the supply of companies. Nonetheless, in different components of the world, correct inhabitants information is difficult to come back by. In sure low- and middle-income nations, the latest census could have been performed a long time in the past or lack correct illustration of susceptible populations. Moreover, estimates between censuses are sometimes fraught with inaccuracies and distant populations could also be totally lacking from official sources. Consequently, uncounted communities could stay exterior the attain of important packages.
To fight this problem, Meta started the process of mapping the world’s population using artificial intelligence and satellite imagery in 2017. Alongside different main inhabitants mapping establishments like Columbia University’s Center for Earth Science Information Network (CIESIN) and WorldPop at the University of Southampton, we now have openly published hundreds of high resolution population maps and datasets. These have been used world wide by governments and nonprofits for social packages starting from the targeting of COVID-19 interventions to the supply of unpolluted water. Because the world’s pure useful resource and vitality calls for scale, correct inhabitants estimates additionally supply vital alternatives to enhance sustainability efforts.
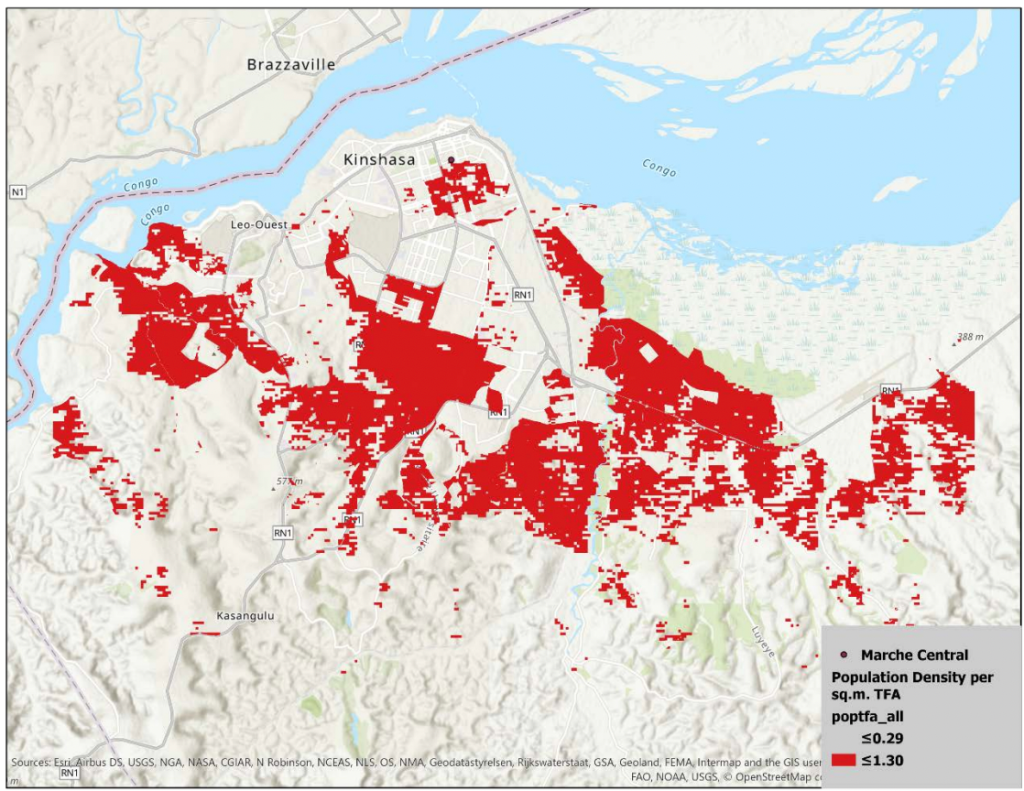
Background on Meta’s AI-powered inhabitants maps
Knowledge for Good’s AI-powered inhabitants maps estimate the variety of individuals residing inside 30-meter grid tiles in practically each nation world wide. These maps leverage pc imaginative and prescient strategies – just like these leveraged to identify objects in photos for the visually impaired – to determine human-made constructions in satellite tv for pc imagery. The outputs of Meta’s AI mannequin are then mixed with inhabitants inventory estimates from CIESIN to approximate the variety of individuals residing in every tile.
Along with complete inhabitants counts, Meta’s inhabitants maps additionally embody demographic breakdowns for teams such because the variety of youngsters beneath 5, girls of reproductive age, youth, and the aged.
AI-powered inhabitants estimates have been scientifically evaluated to be among the many most correct on the planet for mapping inhabitants distribution for quite a lot of geographies and use-cases. For instance, this 2022 paper by researchers at the University of Southampton and University of Ghana in Nature – Scientific Reports compares varied inhabitants density estimates to be used in mapping flooding danger in west Africa. Different research have investigated quite a lot of use-cases resembling mapping landslide risk and malaria eradication; and a variety of nations together with Haiti, Malawi, Madagascar, Nepal, Rwanda, and Thailand.
Open-sourcing coaching information for our AI inhabitants maps
This preliminary set of coaching information consists of virtually 10 million labels for over 126 gigabytes of satellite tv for pc imagery and consists of human labels on these satellite tv for pc imagery patches indicating if a constructing is current. These labels were created on satellite imagery dating from 2011 – 2020; nevertheless, even labels made on older imagery are helpful to coach the following era of machine imaginative and prescient fashions (like Meta’s Segment Anything) to extra precisely determine buildings in a variety of land-cover environments. Along with this primary batch, we plan to launch extra information and code for pc imaginative and prescient coaching sooner or later.
Open sourcing Meta’s coaching information and code permits inhabitants mapping companions like CIESIN and WorldPop to proceed the progress made within the final decade. These instruments cut back improvement prices for analysis items to generate much more correct inhabitants estimates and likewise permits researchers engaged on constructing detection to enhance their strategies, particularly when mixed with newer satellite tv for pc imagery. Future information launched from CIESIN and information collaborations like GRID3 will proceed to push boundaries of spatial decision and accuracy as the results of their work collaborating with many African nations to generate, validate, and use core spatial datasets in help of sustainable improvement.
To higher visualize village settlement areas and calculate service protection, World Imaginative and prescient turned to an progressive dataset developed by Meta’s Knowledge for Good (D4G) and Columbia College’s Middle for Worldwide Earth Science Data Community (CIESIN). The ensuing Excessive Decision Settlement Layer (HRSL) has been a game-changer for visualizing the geography of unpolluted water.
–Allen Hollenbach, Technical Director for World Imaginative and prescient Water and Sanitation
Purposes in sustainable electrification, clear water, and local weather change adaptation
Nonprofit organizations and governments world wide have already leveraged Meta’s AI-powered inhabitants maps for a variety of social impression packages, together with the World Bank’s rural electrification efforts in Somalia and Benin and related efforts in Uganda by the World Resources Institute.
World Vision has additionally used these datasets in accelerating the progress in five-year plans for water and sanitation in locations like Rwanda and Zambia and only recently introduced having reached one million additional Rwandans with clean water utilizing insights from these maps to trace progress in direction of common water protection.
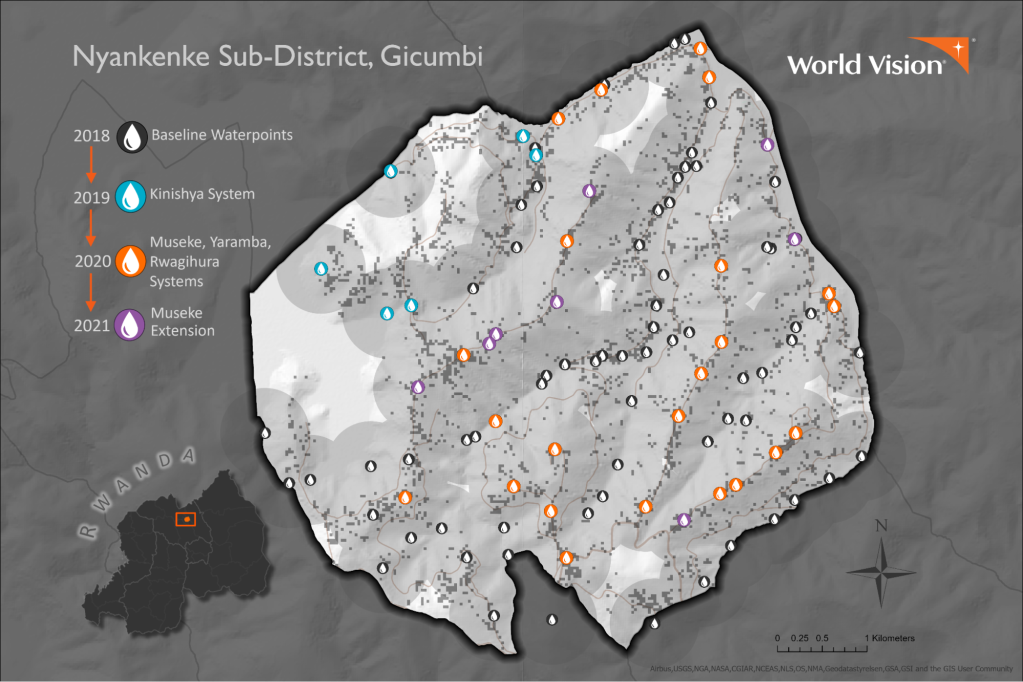
Innovation in international inhabitants mapping is simply potential by the kind of collaboration Meta continues to have with Columbia College and WorldPop and a shared dedication to open supply permits researchers and governments world wide to take part on this course of.
Please go to the Data for Good web site for extra details about Meta’s Knowledge for Good program. And please go to this weblog for extra information about how we protect user privacy in our tools.